We take an information-theoretic approach to identify nonlinear feature redundancies in unsupervised learning. We define a subset of features as sufficiently-informative when the joint entropy of all the input features equals to that of the chosen subset. We argue that the rest of the features are redundant as all the accessible information about the data can be captured from sufficiently-informative features. Next, instead of directly estimating the entropy, we propose a Fourier-based characterization. For that, we develop a novel Fourier expansion on the Boolean cube incorporating correlated random variables. This generalization of the standard Fourier analysis is beyond product probability spaces. Based on our Fourier framework, we propose a measure of redundancy for features in the unsupervised settings. We then, consider a variant of this measure with a search algorithm to reduce its computational complexity as low as with being the number of samples and the number of features. Besides the theoretical justifications, we test our method on various real-world and synthetic datasets. Our numerical results demonstrate that the proposed method outperforms state-of-the-art feature selection techniques.
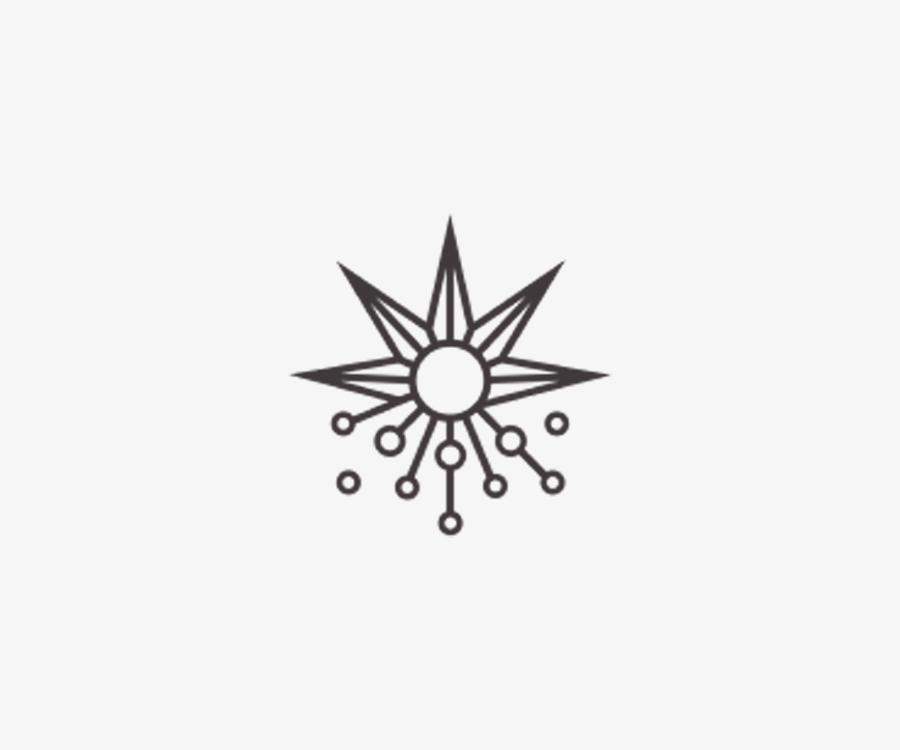