As a research-driven organization, the ability to experiment and prototype rapidly is one of the most important elements of the work we do. We started where most people start, using standard algorithms and experimenting with them. This strategy worked in the beginning because most projects were in the preliminary stages. Over time, these projects started to increase in complexity. There were a plethora of new components added each week. There were new datasets, new metrics, new training mechanisms, new analysis mechanisms added to these projects regularly. Teams grew bigger and more diverse with different functions. And before we knew it our codebases became harder to manage and each new request started taking more time than it should have.
This meant that simple changes in the code required overall context. Think about it as entangling your earphone wires. But this time you had five earpieces instead of two and if you didn’t disentangle it right, you wouldn’t be able to hear anything. As you pull one thread it could tighten knots elsewhere. It may seem like fun in the beginning but it gets harder and frustrating as systems get more complex.
This is an example of a poorly written system.
Philosophically, poorly written software systems are tightly coupled and extending the system for new requirements and reusing the system for new projects within the company becomes a hassle. This creates redundancies. Not just code replication but also duplication in human effort to rewrite existing modules as well as to re-test newly written modules. Researchers and engineers effectively feel that they’ve spent time fixing runtime errors and tests rather than focusing on experiment design and the larger research problem. This also causes barriers to entry when on-boarding new members to the team. But because of the complex nature of these systems, it takes even experienced members longer and longer to add new features.
The goal was to list the principles that are of priority to us as a research institute and design a system based on them. We focused on
- Extensibility/Flexibility – Adding new components should be relatively straightforward and should not require knowledge of the entire system
- Configurability – Machine learning systems differ from traditional software systems in that the requirements are not deterministic and instead involves thorough experimentation where multiple parameters are changed. The goal is to enable such changes via a configuration file without changing code. That way, researchers can run hundreds of different experiments without editing the code at all, not only saving precious time but also reducing the probability of new bugs being introduced!
- Testability – Systems should be easily testable in that different components should be testable independently without knowledge of the entire system.
- Reproducibility – Ability to reproduce past results is of utmost importance to us. As researchers, it is important for us to enable others (including our future selves) to reproduce our results. That not only builds trust in our system but allows others to extend directly on top of our work.
- People Processes – Along with software design principles, it is equally important to create a culture
Researchers at Wadhwani AI strike a balance between conducting novel research and incorporating the research into our solutions. Though they are very closely related, the pathways for both might differ at times and following the above principles has allowed us to strike this balance perfectly.
Every question must have an answer
Once we realized where we were, we took a step back and designed our research codebase from scratch, focusing on what it should look like rather than what it was and how we fix it. My colleague, senior researcher Jigar Doshi and I had regular meetings. It took us two weeks before we zeroed in on a design. We then piloted and implemented this in our anthropometry project. Things started to improve almost immediately. Once word got out about how we had increased efficiency in the anthropometry project, the pest management and TB projects both adopted it. In fact, our COVID-modelling and Cough against Covid projects were imagined with this design in mind. Our design helped the teams in those project build systems faster, and it led to quicker prototypes.
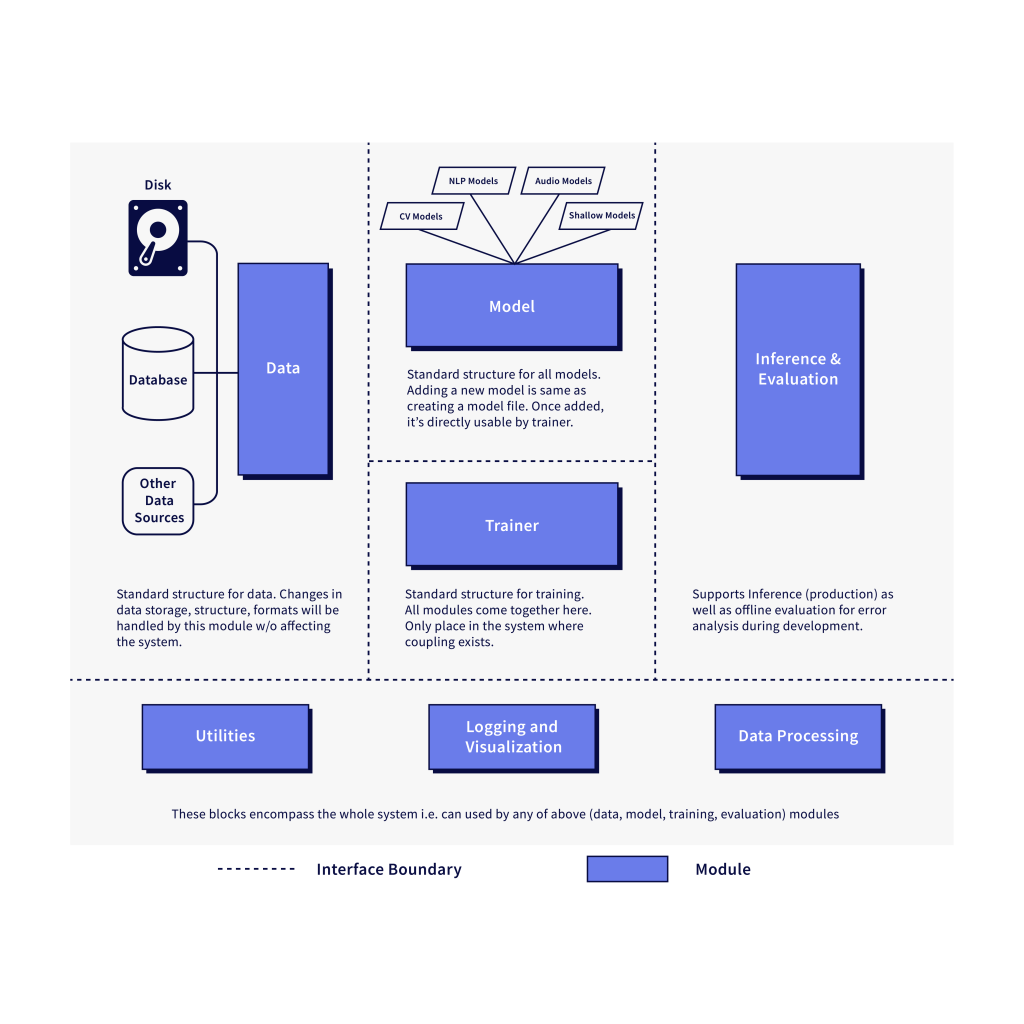
Conceptually, it all hinges on decoupling the various modules by introducing standard interfaces for data, model, trainer, visualization, evaluation and making the interaction between these independent modules standardized. The underlying vision is to make the whole codebase as configurable as possible, and enable running newer experiments (and conduct research) without spending too much time editing code. It will also ensure that the configuration itself does not become too much of a burden. Another important aspect of a well-designed system is the people processes that go along with it. We framed and adopted processes for code review, version control and collaboration both within and across teams.
If I had to simplify this: All we wanted to do was build a system that allows researchers to experiment without spending most of their time on engineering.
Our win
After implementation, we observed increased productivity among our researchers. How? It can be measured.
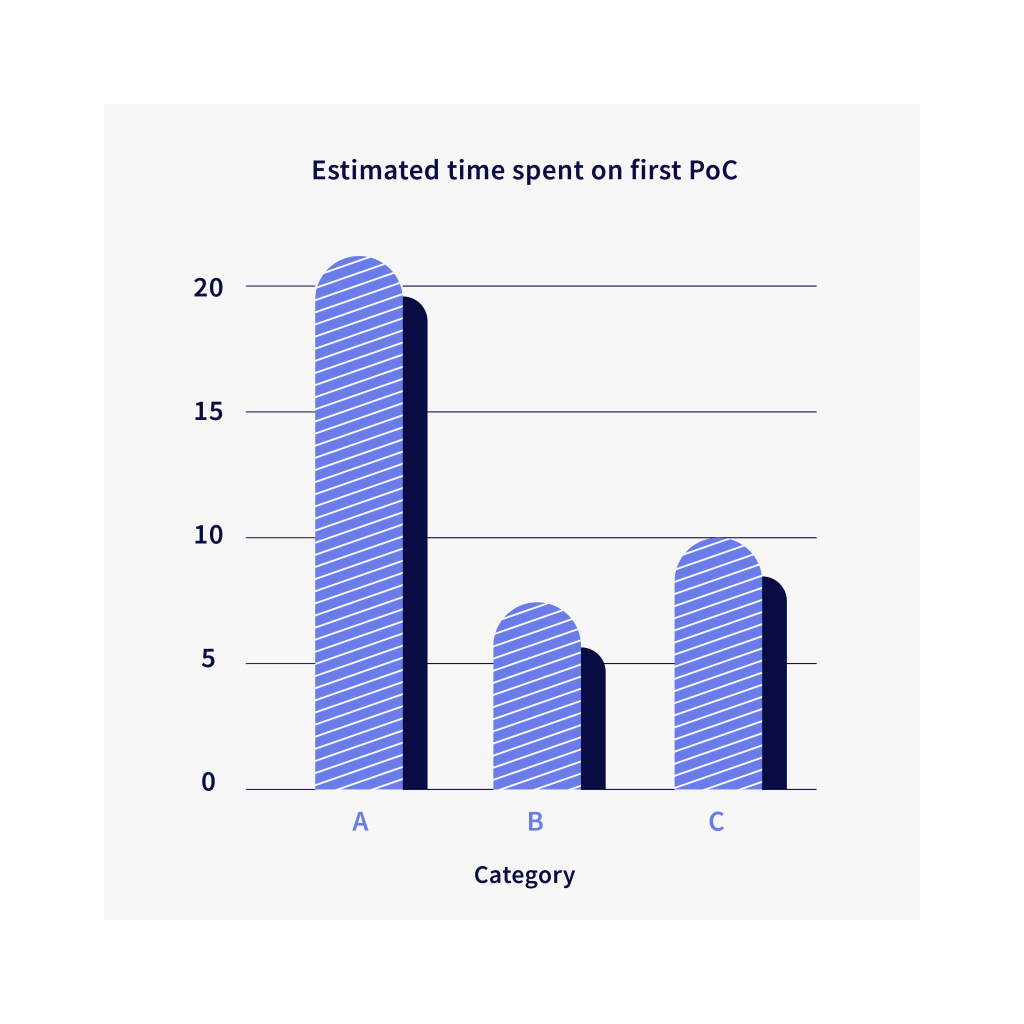
A – Starting from scratch
B – With good design, tailoring older code to new use case
C – Without good design, but individual functionalities from other projects can be borrowed
*Estimates calculated after speaking with our researchers about existing projects.
“The ML code guidelines (in particular by enforcing modularity with respect to data, models and optimisation) make it very easy for a newcomer to quickly get to grips with the codebase and innovate without having to spend weeks understanding the system. As a visiting researcher, I had a very short time to try out numerous ideas, the well-structured codebase helped me rapidly implement and experiment with incremental changes, for example extending a single-frame model to a video-based model with minimal duplication and testing,” Arsha N told me. She is a visiting researcher at Wadhwani Institute for Artificial Intelligence.
Work that used to take about three weeks now takes about one. There is increased reuse of code across sub-projects. There is more rapid experimentation as different configurations of networks, data, other training parameters are all configurable. It means that we create a new config file and that represents a new experiment with new settings (new dataset, a new state of the art model, new training mechanism etc.). This makes life much much easier. What it also does is it makes it easy to onboard new members to the team. A small bonus is that we have found an organic growth to our code guidelines and usage across all of our research projects over the past few months.
And this will only increase with time. We have managed to cut costs, especially when it comes to starting a new project.